AWS Knowledge
Understanding AWS SageMaker Pricing & Cost Management
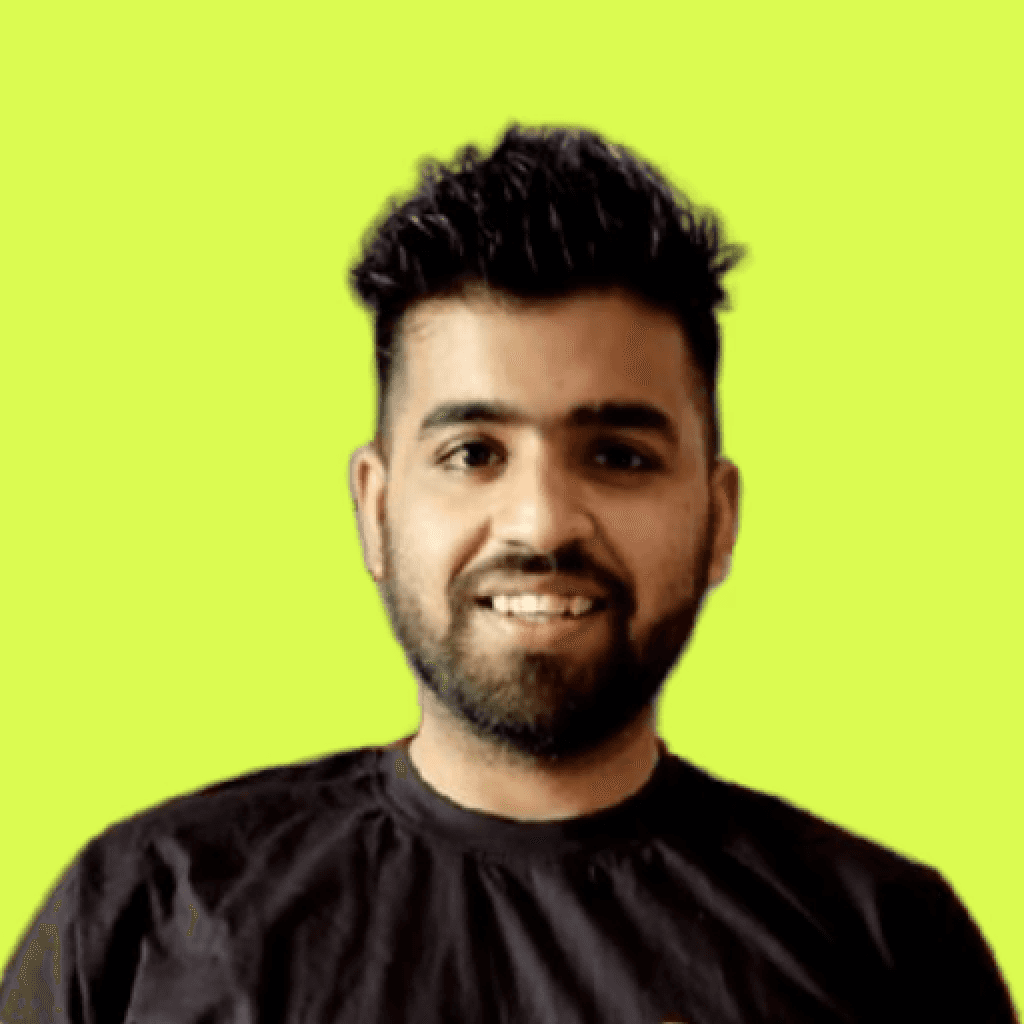
Piyush Kalra
Sep 27, 2024
Today, machine learning is a tool supposed to shape the new business landscape. However, this technology might be quite complex in terms of pricing. Thus, finding the pricing model of AWS SageMaker is very essential for data scientists, cloud developers, or business professionals who aspire to use the service. First, AWS SageMaker, which has gained a significant portion of the market, is very much associated with the services of machine learning and accounts for about 10% of the cloud-based machine learning segment in the global market. This knowledge not only helps in efficient budgeting but also maximizes your investment in this advanced service. In this chapter, we will discuss some of the intricacies of Amazon SageMaker pricing, present cost-cutting strategies, and find some keys to optimizing your usage of this very effective tool.
What is Amazon SageMaker?
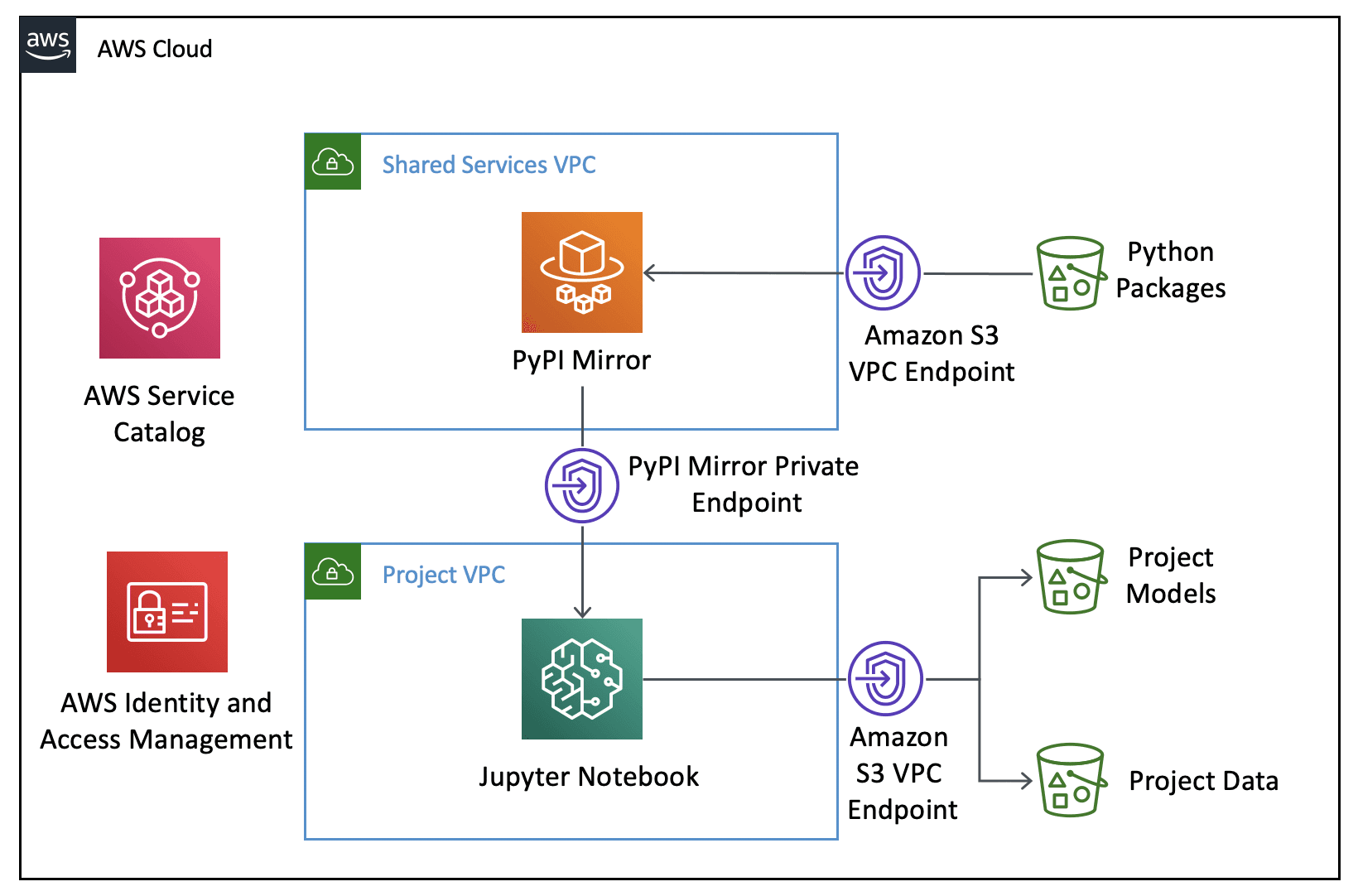
(Image Source: AWS Cloud)
Amazon SageMaker is one of the most popular services among various machine learning services' management. It is a service that allows developers and data scientists to easily build, train and deploy machine learning (ML) models with no complexity involved. Because of streamlining each pixel of the machine learning process, high-grade models can be developed with the assistance of SageMaker. This provision has proven to be quite advantageous since one can rent Studio Classic for as low as $0.05 per hour on a pay-as-you-go model. SageMaker is equally beneficial in that it charges by the second for any period of resource utilization with no minimum charge. This flexible pricing can suit your case, whether it is a new model's testing or a ML solution's scaling. New AWS users can explore SageMaker with a free tier to start without initial costs.
Functionality of Amazon SageMaker
For any users of the system whose main problem is model deployment, SageMaker has enormous potential that simplifies the entire ML workflow particularly in the model preparation, building, training and finally, the deployment of models. Apart from offering the structure for the modeling process, SageMaker provides other basic ingredients of ML such as Jupyter notebook instances for data exploration, built-in algorithms and frameworks, automatic model tuning and one-click deployment functionality. SageMaker is a modular ML software that can either be used as a whole or in parts.
Key Components of SageMaker
SageMaker Ground Truth: Enables continuous contribution of human input to ensure the development of accurate training datasets in the ML process. It connects with human labelers through Amazon Mechanical Turk, external sources or one’s own workforce to create data for training. Moreover, Ground Truth uses ML for the pre-labeling of data which saves time and storage costs by offering suggestions of proposed labels for foreign review.
SageMaker Studio: The said product is a cloud-based development environment designed for ML activities and integrated all in one web interface that allows users to perform coding, share usage and aesthetics of the application, management of experiments, presentation of the findings and testing of the application. With Sagemaker studio, machine learning models can be built, trained, debugged, deployed, and monitored.
SageMaker Model Monitor: Checks the performance of the deployed ML models periodically. It seeks to determine if there has been any abnormalities in the data being used , the operational model or any impacting parameters related to model operations and sends out alerts for other actions to be taken to correct the situation. This maintains accurate models including model performance and operational performance. AWS is assisted by a model monitoring system which is a module in SageMaker specifically designed to automatically track the model performance and its behavior to check for any possible drifts especially if there have been changes in data sequence.
AWS SageMaker Pricing Models
AWS SageMaker has provided a broad array of options with regard to furthering these services. You only pay according to your needs, as there are two main pricing mechanisms: the On-Demand Pricing, which has no baseline fees and no retention agreements, and the SageMaker Savings Plans, where its simpler rules of using credits in accordance to usage is balanced against the steady usage charges amount one commits to. Evaluating these models, you can see their advantages and how you can apply them effectively on your ML projects.
Free Tier
Free tier of Amazon SageMaker can be tried out. The new users can access Amazon SageMaker free of charge as part of the AWS Free Tier from the first month in which they first create a SageMaker resource – resulting in a free trial of Amazon SageMaker. The free tier also provides 250 hours of usage of ml.t3.medium instance types on Studio notebooks or notebook instances, 250 hours of usage of RSession app, 25 hours of usage of Data Wrangler, 10 million Feature Store write/read units, 50 hours of training on specified instance type, 300 hours usage of TensorBoard, 125 hours real-time inference usage, 150,000 seconds serverless inference, 160 hours per month Canvas session time and 50 hours of usage of HyperPod for the first two months.
On-Demand Pricing
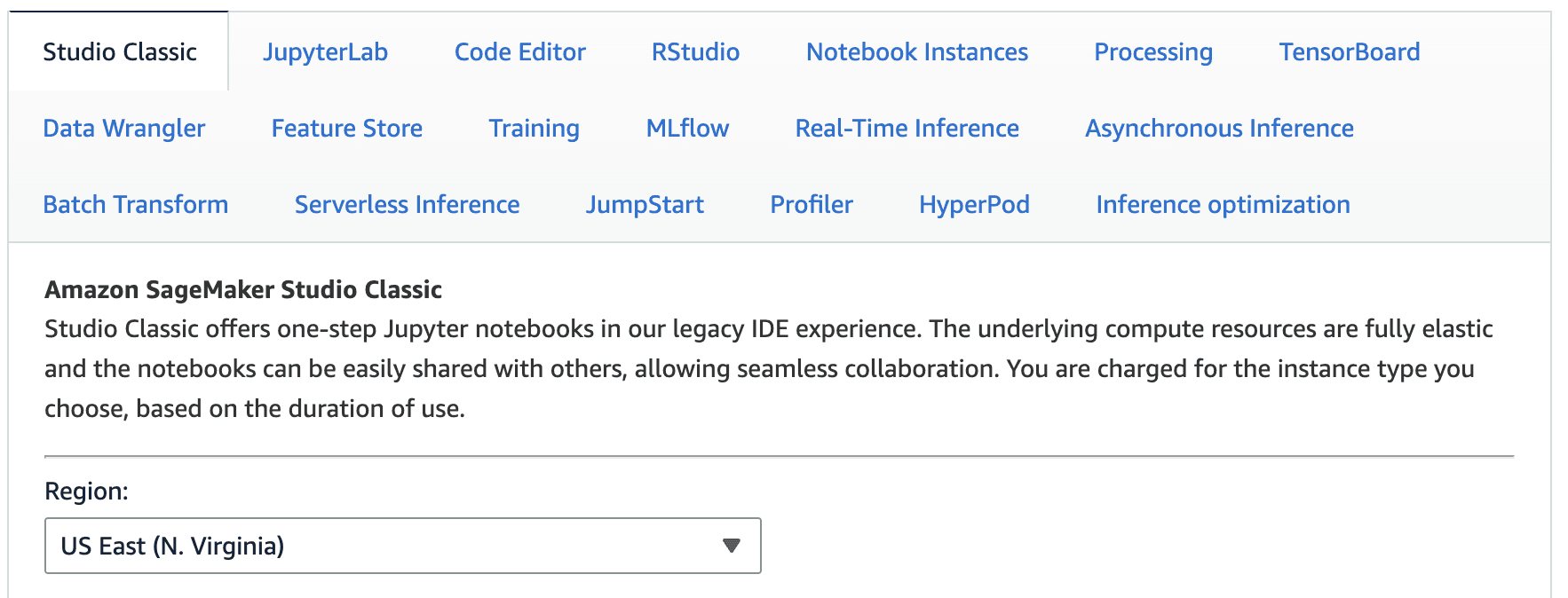
On-demand pricing provides options to clients that they don’t have to commit for a long time. You only pay for what you use according to how long you took the resources, charged by the second. This model best fits the use cases that have an unstable workload or even customers that are new to AWS SageMaker. It promotes innovation with crazy ideas since no one is afraid to try due to funding issues. On-demand pricing is best suited for infrequent machine learning workloads or whenever there is a need for an additional computing capacity.
Savings Plans
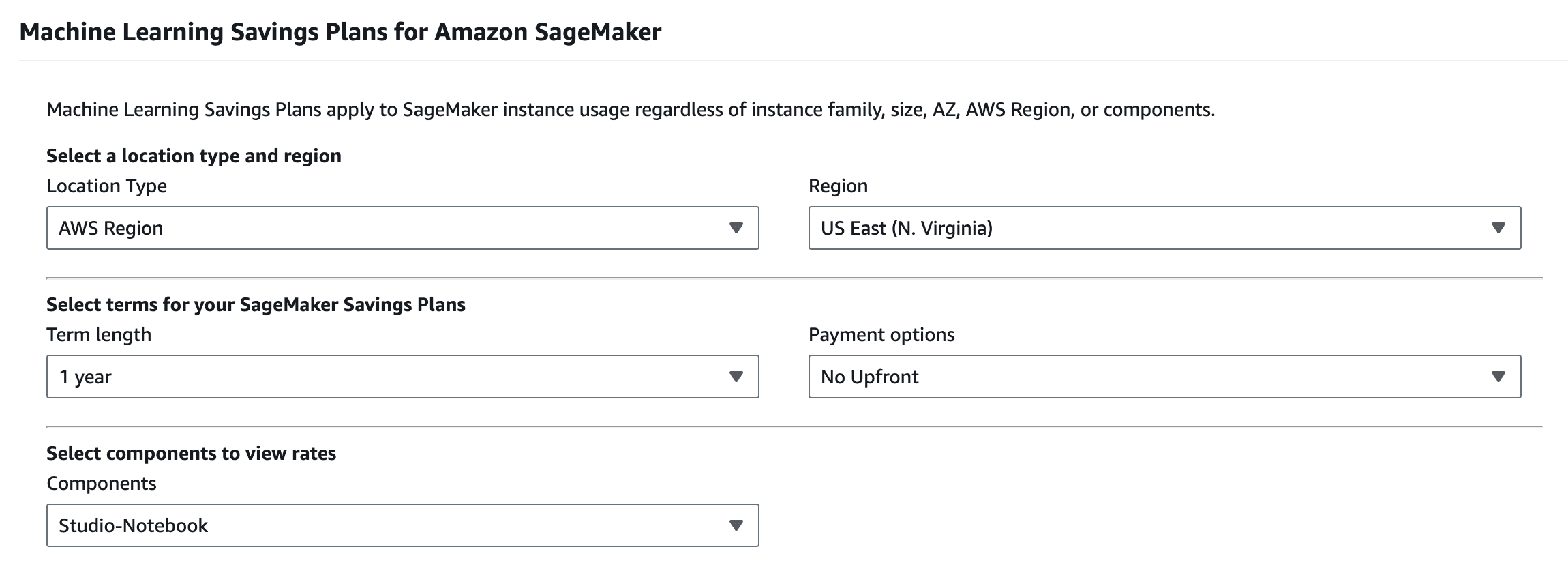
For those ready to commit, AWS SageMaker Savings Plans provide huge discounts of usage in exchange for one or three-year commitment for steady use. Such plans give a maximum of 64 % in savings compared to the on-demand pricing. It is recommended to teams with steady and predictable workload patterns making it easier to plan and monitor budgets. Because of a savings plan, the total cost of ownership is less than that of the use of AWS SageMaker with the same high level of usage.
More Pricing Elements
Amazon SageMaker pricing is also affected by several other factors that may not be so apparent. These factors include the form and amount, in the case of instances that are being used, storage space, and the amount charged for data movements as well as other services such as SageMaker Studio or Model Monitor. It is very important to select an instance type which is appropriate to the workload demand as it promotes significant cost savings. Also, for those who have to use such services, the cost for the different types of storage and for the information retrieval may also increase costs meaning customers should always be careful on the use of transfer and data storage. Knowing these factors is beneficial when it comes to working out more budget structures and how to allocate the necessary resources. You can use SageMaker AWS Calculator to understand the pricing better.
Cost Management Strategies
When managing AWS SageMaker expenses, it takes knowing that the costs can not just be avoided but properly scoped out. Here are some strategies to help you control expenses:
Monitoring Usage and Spending
To make sure that the management of costs is on top, AWS Cost Explorer is an indispensable tool. For instance, countries with a more expansive budget can set up alerts and then based on those alerts, correct the spending to meet budget targets. Tracking expenses regularly helps in discouraging unnecessary costs and resources are utilized optimally. Thanks to Cost Explorer, usage statistics can be analyzed to detect trends, predict future costs, and determine reasonable amounts of spending to ensure profits.
Optimizing Resource Allocation
The appropriate use of instances is very crucial in resource allocation. For instance, assess your workload’s requirements and select instances such as T3 or M5 which correspond to your performance requirements. Resources should be adjusted in accordance with the necessity, this can help to avert unnecessary checks. It is also possible to add auto scaling capabilities to allow automatic operating environment optimization based on the current activities thereby minimizing costs.
Automated Tools Usage
AWS Budgets and cost optimization tools are very helpful in improving cost management processes. They help increase efficiency by offering management tools where users have a chance to manage costs through action plans focused on minimizing costs. One instance would be the employment of optimal methods in cost gauging such as devising measures such as automatic notifications and reporting. It is possible to manage costs, control wastage and cost overruns with minimal time with the help of automated tools and systems.
Cost Optimization Best Practices
Achieving the best possible outcome with the least possible cost implies that one must also use particular cost-efficient practices that have been designed with AWS SageMaker in mind. Here are some key strategies:
Regular Review of Usage Patterns
Various improvements can be made through review of usage information after some time. With historical data in hand, you can make necessary changes and fine-tune resource allocation. It guarantees that only the best solutions that are cost-efficient are employed at any one point in time. The use of trends in prediction to meet future needs can be assisted in order to mitigate the costs instead due to the need for change. AWS Cost Explorer can greatly facilitate the evaluation and analysis of trends.
Using Spot Instances
Spot instances are a viable idea to save on costs as they lease EC2 recyclable resources. These instances are mainly applicable to fault tolerant and flexible applications. Using spot instances in your architecture will reduce costs without affecting the quality of services. Applications that are mainly data processing and batch jobs that span over a long period for instance such applications do not require immediate attention and thus interruptions are not very detrimental. This can be particularly cost-effective when deploying spot instances for batch data processing.
Training Job Optimization
Training jobs optimization is very important as far as costs are concerned. If there is no need for low latency, consider using batch processing to cut down computational costs. Optimal data preparation and hyper parameter tuning can save some bucks as well. With a careful strategy in the training schedule and the use of parallelism, the processes can be completed in a relatively short time and at minimal costs. Optimization of training jobs can be done effectively with tools such as SageMaker Debugger and SageMaker Autopilot.
Use Pump to Cut Your AWS Bill by 60%
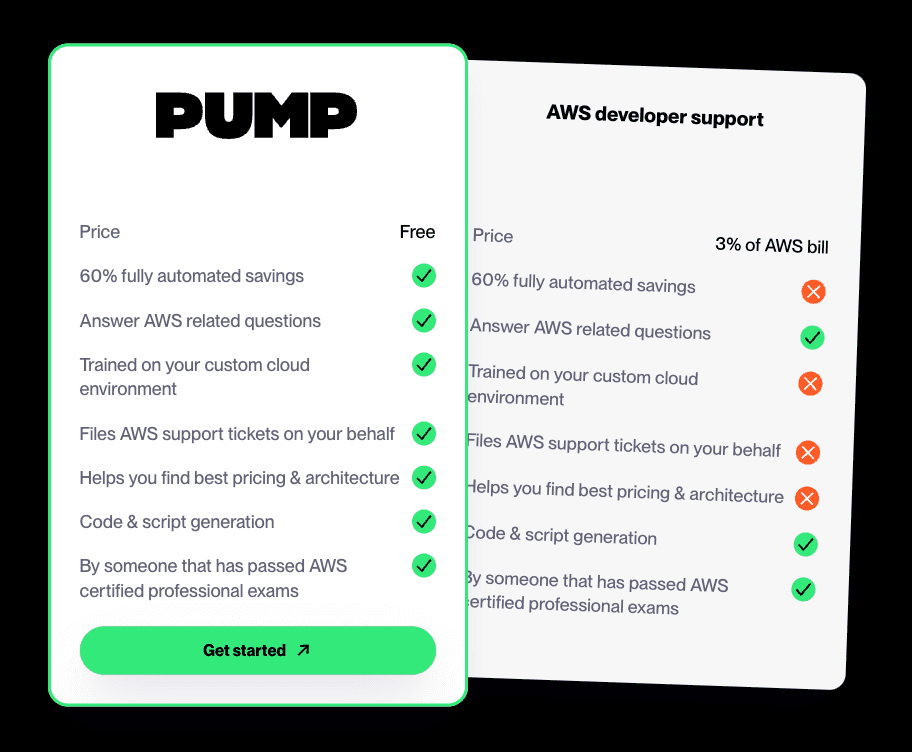
Slash your AWS spending by up to 60 percent for early-stage startups or completely free. Since no other company has built in AI automated savings on 12 AWS services, including SageMaker, Pump can be integrated into your cost management strategy and enjoy great savings without service compromise.
Imagine AWS SageMaker expenses reaching $100. With Pump, those costs could shrink to just $42. Our cost-saving strategies lead to similar reductions across other AWS services, making your cloud operations more efficient and budget-friendly.
Case Studies and Examples
Real-world examples demonstrate the effectiveness of cost management strategies in optimizing AWS SageMaker expenses, leading to significant cost savings. Companies like Articul8 AI, Recursal AI and Rocket Mortgage have reduced unnecessary expenditures and improved efficiency through strategic practices.
Amazon SageMaker HyperPod has significantly improved our resource management, boosting productivity by up to 35% and rapidly scaling our GenAI operations. The new Amazon EKS support seamlessly integrates with our existing Kubernetes training pipelines, streamlining operations and enhancing our GenAI platform for customers.
SageMaker HyperPod streamlines our processes, utilizing resiliency features to recover training jobs from checkpoints during hardware failures. With diverse workloads and Kubernetes integration, Amazon EKS with HyperPod fits perfectly into our cluster.
Nathan Wilce, Infrastructure/Data Lead, Recursal
Partnering with AWS, Rocket Mortgage is leading AI integration in homeownership. SageMaker enhances our machine learning operations, boosting efficiency and precision. The Pipelines visual editor allows rapid performance assessments, enabling our data science teams to focus on innovation.
Shawn Malhotra, Chief Technology Officer of Rocket Companies
Conclusion
Because AWS SageMaker is such an advanced and cutting edge technology, understanding its pricing and implementing strategies for the appropriate utilization of resources will go a long way in helping you reap high returns from your investment in this machine learning service. By putting into practice the recommendations and muscle memory of this guide, resource usage and expenditure can be optimized.
No matter if you are a beginner with AWS SageMaker or want to expand further in your existing setup, these steps will help you to achieve affordable solutions for machine learning projects.
Join Pump for Free
If you found this post interesting, consider checking out Pump, which can save you up to 60% off AWS for early-stage startups, and it’s completely free (yes, that's right!). Pump has tailor-made solutions to take you in control of your AWS and GCP spend in an effective way. So, are you ready to take charge of cloud expenses and maximize the most from your investment in AWS? Learn more here.