One technology that’s been catching a lot of eyes is Google BigQuery. Famous for its scalability and power in data analytics, Google BigQuery is a cloud data warehouse that easily handles massive datasets. However, in order for startups to take full advantage of it while avoiding going bankrupt, it is important for them to know how the service’s pricing model works.
Now, in this blog post, we’re here to educate startups, data analysts and small company owners on how Google BigQuery pricing works. You’ll be taken through the elements that make up the pricing policy, how one is able to economize on the expenses, and also practical suggestions on how to use the BigQuery without incurring excessive costs.
What does Google BigQuery do?
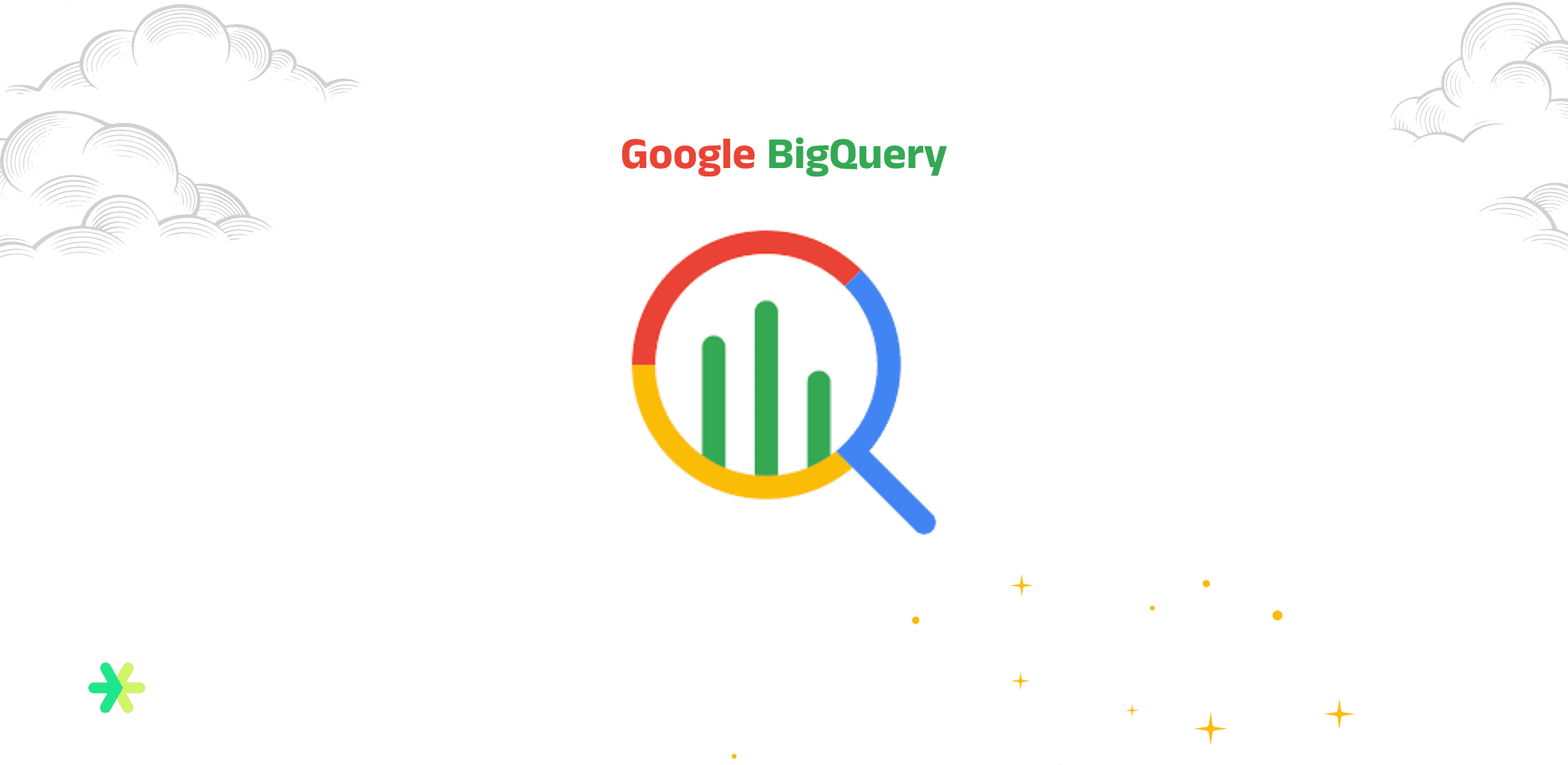
When it comes to handling data in the business context, Google BigQuery presents itself to be the solution for one of the biggest data management problems. Thanks to its cloud-and-serverless, fully managed data warehouse system, BigQuery is made in such a way that any type of business can afford and access data analyzing at a large scale. It has an architecture that can naturally support data regardless of size and processes high levels of queries in seconds. This is crucial for firms that want to get real-time insights from their data. Strategic cost minimization techniques can help cut expenditures for data generation and management by 30%.
It is important to understand the pricing alternatives provided and formulate pricing tactics which meet the business objectives in order to enhance the usage of BigQuery. In the current day and age, where optimizing costs should be an objective for every organization, BigQuery enables these organizations to document and analyze data at an efficient and lower cost.
Key Components of Pricing
There are two factors that information or end-users for using Google BigQuery will be charged. Which are storage and analysis:
Storage Costs
BigQuery storage costs are based on the amount of data stored by a user which includes both active and long-term storage. These costs are important to know in order to be effective in data budget allocation.
Active storage: Updated tablets and partitions within the last 90 days are in this data storage category. It has the cost of $0.02 per GB active. More frequently accessed or changed data is stored in active storage. In every given month the first 10 GB are free; hence a 200GB table for a single month will cost $4.
Long-term storage: If there is no activity on the updates for a period of 90 days, then the costs of storage reduce further by 50% thus making it to be 0.01 per GB per month. It is ideal for storage of archived data which do not need to be accessed regularly. A table of 200GB costs $2 monthly in long term storage. If any updates are done, it is switched to active storage and the 90 day period is lost.
There is no difference in performance, data security or availability for all data regardless of the classification of being long term storage or active storage. This ensures that businesses are able to cut costs but ensure the caution of data is in place and is available when needed.
BigQuery Cost Per 1 GB
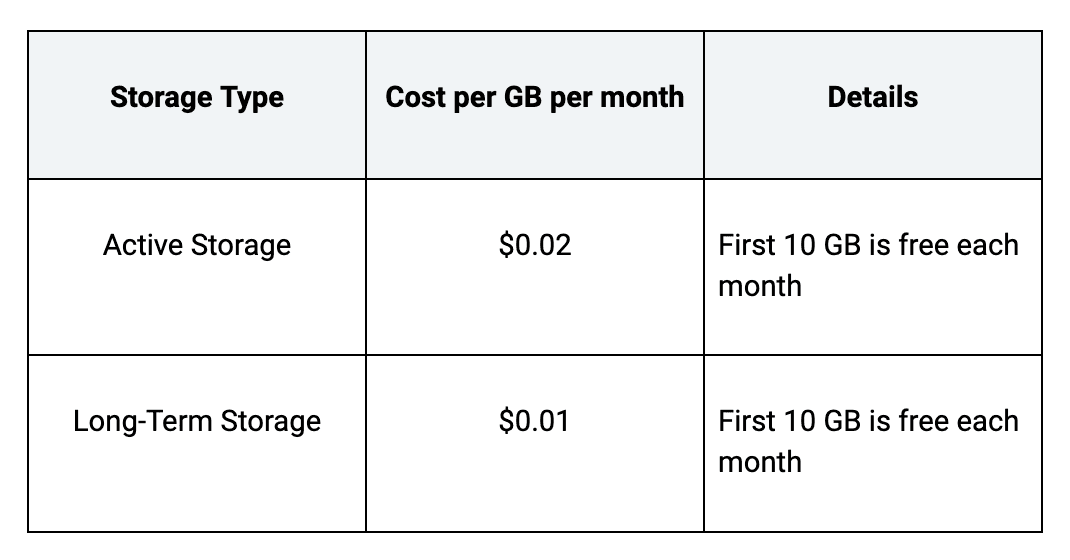
For Example:
When it comes to storage costs there are charges that are incurred for data in tables, temporary session tables and temporary multi-statement tables, but not for temporary cached query result tables.
Data stored is calculated in dollars per MiB per second according to the size of the columns.
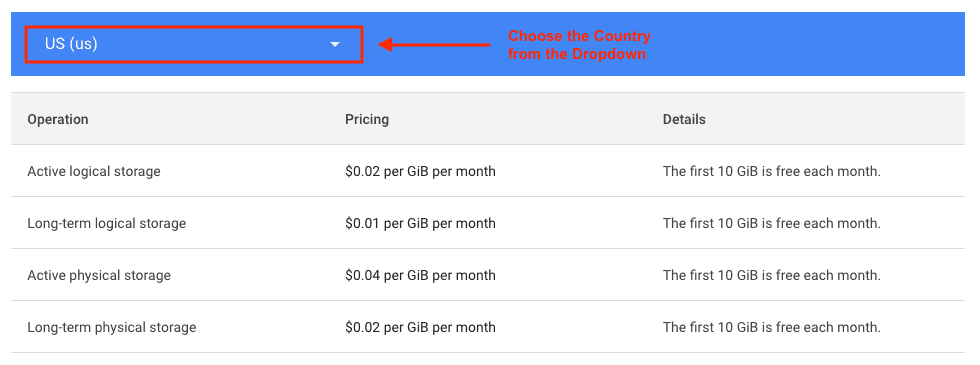
For instance, in the region of us-central1:
512 MiB for half a month costs $0.00575 USD
100 GiB for half a month costs $1.15 USD
1 TiB for a full month costs $23.552 USD
Storage is charged in GiB months that uses base 16 where 1 GiB is given by 230 (1,024 MiB) and a tebibyte of storage is given by 240 (1,024 GiB).
Once the data has not been edited or deleted for 90 days or more, it is treated as long-term data and charged as such, although there is still no compromise on performance or functionality. There is virtually no chargeable activity on the unmodified partition. However, each partition of the partitioned table is treated independently and the long-term rates apply from the 90 day onwards.
When one makes modifications to a table, the price for the services offered is reverted to the standard charges, thereby starting the timer set for 90 days again. The timer as well as the status of 90 days can be reset by performing certain actions such as loading or pasting data into the table, writing the results of queries into a table, performing data manipulation or data definition language or streaming data into the table. But there are certain things that do not reset the timer such as querying a table, creating a view, sending information outside, copying a table, and creating patches for or updating some portion of a table resource.
In this case, the long term pricing would apply to the storage of the BigQuery only whereas external data sources will not be affected.
Analysis Costs
These costs are incurred for making calls to the data in Big Query through different means such as user-defined functions, SQL queries among other scripts. The major factor of concern is the amount of data that is being processed. There are two main options under google big query’s pricing model which include:
On-demand pricing: This describes the mechanism where queries that read and write data are billed, subject to an allowance for a first terabyte of data processed per calendar month. This model currently boasts a low barrier to entry into important levels of handling big data analytics. If you query for a large amount of data and it was not successful, you will not incur any charges for the number of bytes handled.
For example, if the project has 1.5 terabytes in January, only 0.5 terabytes is charged because the first terabyte is free.
Normally, subscription based pricing allows a fair degree of access up to about 2,000 overlapping slots, on average calculated across all queries in a single project. In some instances such as with BigQuery running smaller queries, the service may go beyond this limit temporarily; however, a customer may have less slots available during peak demand for on demand capacity within a particular area.
Flat-rate pricing: With flat-rate compute pricing, you can manage your costs for queries in BigQuery because you purchase data processing capacity in the form of slots rather than paying for data processed. This flat rate can be appealing to users seeking predictable covered queries (BigQuery ML, DML, DDL), but not storage or BI Engine expenses. Minimum of 100 slots required, which can be purchased on a flex, monthly or yearly basis. Slots are region-locked and shared among the organization. 60-second flex slots have been allowed for short-term use. BigQuery Omni has the same price list for AWS and Azure as well. You also receive free BI Engine capacity of a maximum of 100 GiB in addition to slot commitments.
For example, If you are a frequent BigQuery user and would like to pay the same amount every month, you can purchase a fixed number of slots. It will also predict how much you will pay each month regardless of how much you query.
Google's New Pricing Models
Google BigQuery has rolled out new pricing last year with 3 new editions Standard, Enterprise and Enterprise Plus which provides Google BigQuery its users with added flexibility and reliability.
Standard Edition: Most suited for ad-hoc analysis, development and testing workloads as it is the cheapest. It includes baseline slots features such as high speed analytics, serverless data architecture and machine learning capabilities. It is appropriate for small and medium scale companies that perform data analytics without sophisticated architectural needs. Cost effectiveness in terms of small workloads as well as availability of baseline slots features of Bigquery are its main advantages.
Example pricing:
Pay as you go: $0.04 per slot hour, billed per second with a 1-minute minimum and no commitment.
Enterprise Edition: It has enhanced governance and security features for customers with complex regulatory requirements. It is best suited for enterprises with large amounts of sensitive data because it includes advanced data management and machine learning features. Some key advantages include strong security and governance features as well as advanced data management capabilities.
Example pricing:
Pay as you go: $0.06 per slot hour, billed per second with a 1-minute minimum
1 yr commit: $0.048 per slot hour, billed for 1 year
3 yr commit: $0.036 per slot hour, billed for 3 years
Enterprise Plus Edition: With this edition, users get all functionalities of the previous editions while gaining extra functionalities for mission critical workload. This is ideal for customers who need high levels of uptime, high availability and high levels of recoverability. Additionally, it provides superior advanced support add ons such as 24 hour standby support and provision of a dedicated technical account manager.
Example pricing:
Pay as you go: $0.1 per slot hour, billed per second with a 1-minute minimum.
1 yr commit: $0.08 per slot hour, billed for 1 year.
3 yr commit: $0.06 per slot hour, billed for 3 years.
Cost Optimization Strategies for Startups
Efficient Query Practices
For normalizing costs efficient querying is important. Don't use "SELECT" statements as this will always require a scan of the whole data set. Only column names should be entered, and a "WHERE" clause used, in a move to minimize the amount of scanned bytes thus reducing query costs.
Data Partitioning and Clustering
Partitioning your data without overloading it with unnecessary details by criteria such as dates serves to cut down costs, as it reduces the amount of data queried. Clustering further improves performance by organizing data according to its field based on some specifics for faster query answering while also lowering costs
Monitoring and Reviewing Usage
You have to check your BigQuery usage and costs on a regular basis using the available tools. Regular reviews will go a long way in optimization as it will look into use of cost effective options. Refer to our article, we have explained the strategies for performance monitoring and optimization.
Practical Tips for Startups
Utilizing Free Tier Options
Startups can make use of the Bigquery free tier plan, which gives 10GB free storage every month, as well as the first TB free of charge for queries processed. Take advantage of these to ensure that you do not incur too much costs when you are starting up and as you expand.
Budget and Projecting Costs
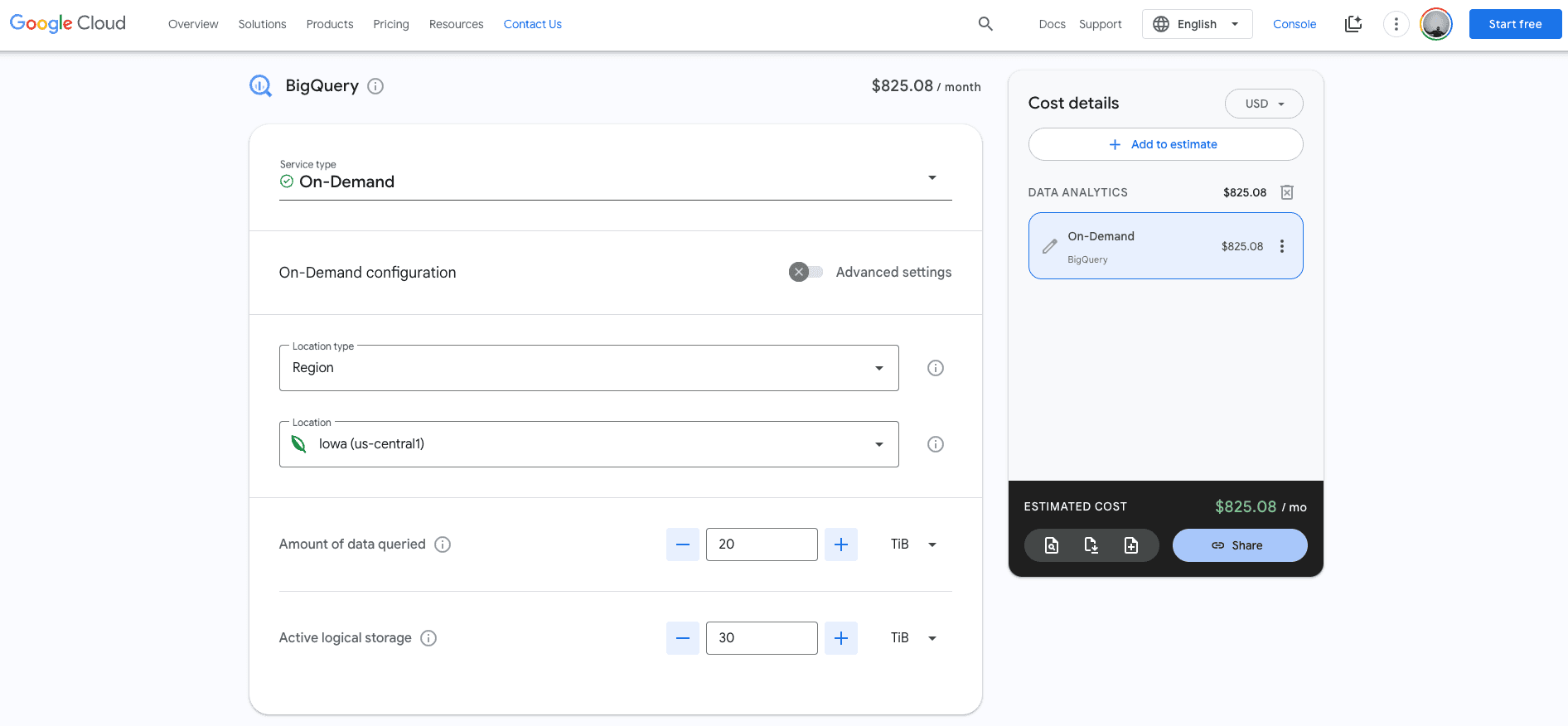
It is important for startups to accurately budget. It is necessary to estimate how much will be spent on hosting and requesting data every month so that the funds are appropriately budgeted. The GCP Pricing Calculator can come in handy when projecting costs, which aids in financial planning.
Conclusion
Startups can take advantage of Google BigQuery’s analytics infrastructure, however, they need to be keen on its expenditure zone as it is one of the main concepts in optimizing its use. Startups can use BigQuery without incurring excessive costs by using effective query techniques, taking advantage of free-tier offerings, and using Google's tools. Remain alert, and control your expenditure while practicing recommended procedures to manage the cost of your data.
Join Pump for Free
If you found this post interesting, consider checking out Pump, which can save you up to 60% off AWS for early-stage startups, and it’s completely free (yes, that's right!). Pump has tailor-made solutions to take you in control of your AWS and GCP spend in an effective way. So, are you ready to take charge of cloud expenses and maximize the most from your investment in AWS? Learn more here.